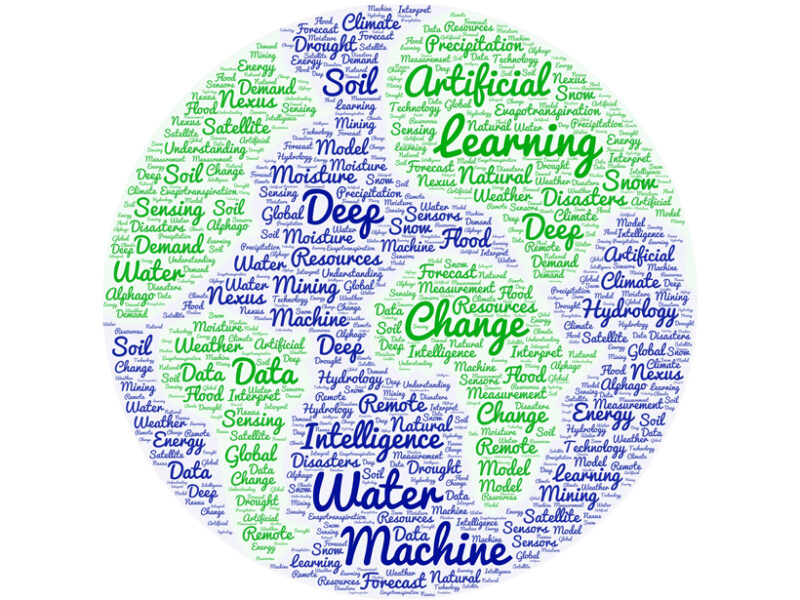
In popular culture, Artificial Intelligence (AI) often refers to machines that can perform any intellectual task that humans can. Such machines are heavily romanticized and are still very far from becoming a reality. However, weak (or narrow) AIs, algorithms that are designed to perform a specific task, have shown a formidable intellectual prowess that surpasses human capabilities in certain tasks. These machines must have integrative decision-making capability based on what they receive and what they predict would happen.
Powered by deep learning, the performance of recent AIs has improved dramatically, and new solutions have emerged across various fieldsUnderneath their recent success, deep learning (DL) often serves as a predictive tool by modeling relationships between inputs and their outcomes [LeCun et al., 2015]. DL helps AI anticipate consequences of its actions, learn features and make decisions. Powered by DL, the performance of recent AIs has improved dramatically, and new solutions have emerged across various fields in a “Cambrian explosion” fashion, including medical diagnosis, natural language processing, and engineering designs.
Take, for example, AlphaGo, the AI that famously defeated world champions at the ancient game “Go.” It utilizes a DL network to identify promising moves and another network to assess the relative strengths of possible moves [Silver et al., 2016]. Both are deep networks that succeed in modeling highly complex mappings in a game with a tree search complexity greater than the number of atoms in the known universe.
Given DL’s success in other disciplines, DL could contribute to a wide spectrum of practical water issues such as drought and flood forecasting, extreme climate event identification and climate prediction, water demand projection, global monitoring with satellite remote-sensing, the water-food-energy nexus, and more. Past experiences indicate that deep learning is much more effective and robust than earlier-generation machine learning methods for many problems [Baldi et al., 2014; Tao et al., 2016; Fang et al., 2017].
A significant advantage of DL for science is that it can be a highly-efficient scientific explorer that is relatively unconstrained by human pre-conceptions. Specifically, we can ask what kind of relationship a deep network has found and whether it identified connections that we overlooked. The Go community already refers to AlphaGo as a teacher and requests its assistance in human understanding of the game. Due to its ability to automatically extract features from data, DL offers a fresh and unique opportunity to detect these relationships, interpret their meanings and expand our knowledge. However, to better exploit the potential of this technology hydrologists will need to embrace deep learning and to collaborate with computer scientists.
Perhaps one day DL will become an inalienable component of the hydrologic discipline itselfWhere there are big data, DL could become an indispensable tool in hydrology. Perhaps one day DL will become an inalienable component of the hydrologic discipline itself, just as Bayesian statistics or numerical solutions to differential equations have. Currently, we are still in the early “value discovery” stage of DL development in hydrologic science.
Moving forward, deep learning has considerable potential to improve process-based models. Process-based models require human pre-conceptions of the physical systems, and they can elucidate causes and effects and bridge data gaps. Deep learning and process-based models clearly complement each other and can have synergies [Ganguly et al., 2014]. More novel approaches need to be developed in the context of big yet unbalanced data, complex and trans-disciplinary process-based models, and observational uncertainty, to explore how deep learning can be used to advance mechanistic modeling in the hydrologic sciences.
To explore and discuss recent developments, Water Resources Research has just issued a call for papers for a new special issue on Big Data & Machine Learning in Water Sciences: Recent Progress and Their Use in Advancing Science, and deep learning is one of the solicited topics. We look forward to having this debate within the hydrology community and beyond.
—Chaopeng Shen, Associate Editor, Water Resources Research; and Civil and Environmental Engineering, Pennsylvania State University; email: cshen@engr.psu.edu
The post Deep Learning: A Next-Generation Big-Data Approach for Hydrology appeared first on Eos.
from Eos https://eos.org/editors-vox/deep-learning-a-next-generation-big-data-approach-for-hydrology?utm_source=rss&utm_medium=rss&utm_content=deep-learning-a-next-generation-big-data-approach-for-hydrology
via IFTTT