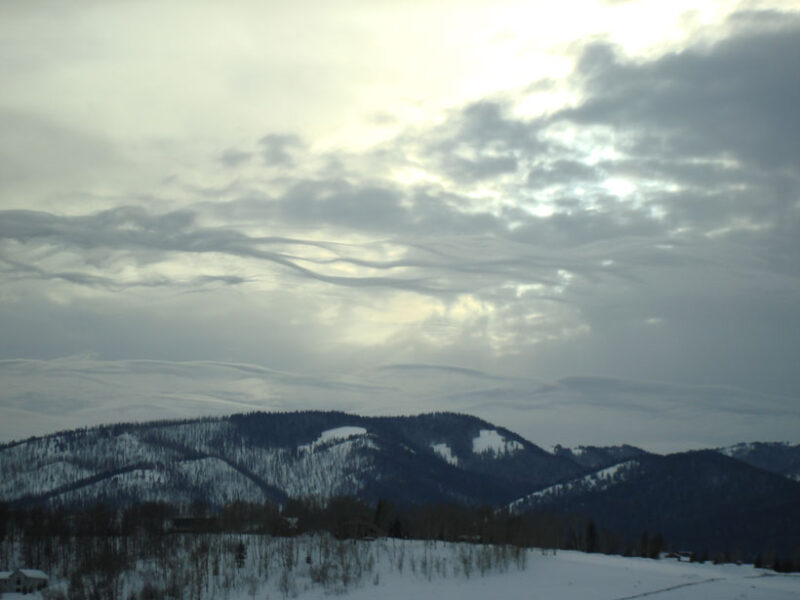
Mathematical models that simulate Earth’s climate are essential for predicting future climate change. However, even today’s most sophisticated Earth system models suffer from uncertainties that stem from the difficulty of simulating small-scale or complex processes, such as raindrop formation and carbon uptake by plants.
Novel computational tools may hold the potential to address these uncertainties. In a new paper, Schneider et al. outline a blueprint for a next-generation climate model that would employ advancements in data assimilation and machine learning techniques to learn continuously from real-world observations and high-resolution simulations.
This approach gets at the heart of the uncertainty that plagues today’s models: a mathematical technique known as parameterization. Earth system models integrate atmospheric, oceanic, chemical, and biological processes, many of which are too complex or occur at scales too small to simulate directly (e.g., formation of individual clouds). Parameterization allows for simplified simulation (e.g., a statistical description of cloudiness) based on underlying conditions, or parameters.
However, underlying parameters and their precise effects are often uncertain, leading to insufficient parameterization and overall model uncertainty. For instance, models with different parameterization strategies give very different estimates of the amount of carbon dioxide in the atmosphere necessary to raise Earth’s surface temperature by 2°C—with critical implications for policy decisions.
To improve parameterization, the researchers propose developing Earth system models that learn from the rich data sets now available (thanks, in large part, to satellite observations) and high-resolution simulations on local scales. Past models have incorporated such information, but the new model could employ modern computational capabilities, including machine learning techniques, to harness and learn from diverse data. This capability would enable a model to continuously update and improve parameterization approaches on the fly, with the potential to improve climate predictions and short-term weather forecasts.
Challenges to this approach include the need for innovation in machine learning algorithms and balancing the computational cost of high-resolution simulations with the value of the information they provide. Nonetheless, the researchers believe a new computational approach to climate modeling can and should start now. (Geophysical Research Letters, https://doi.org/10.1002/2017GL076101, 2017)
—Sarah Stanley, Freelance Writer
The post Next-Generation Climate Models Could Learn, Improve on the Fly appeared first on Eos.
from Eos https://eos.org/research-spotlights/next-generation-climate-models-could-learn-improve-on-the-fly?utm_source=rss&utm_medium=rss&utm_content=next-generation-climate-models-could-learn-improve-on-the-fly
via IFTTT