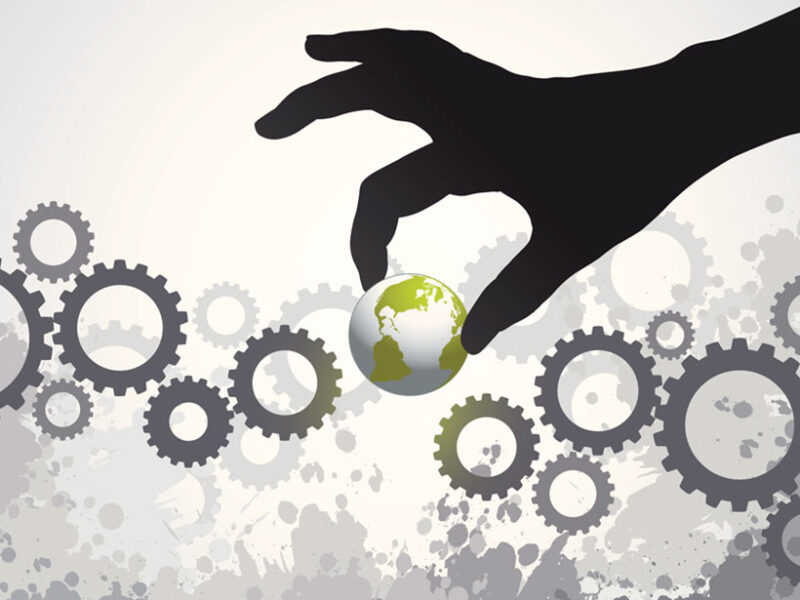
Model uncertainty is one of the biggest challenges we face in Earth system science, yet comparatively little effort is devoted to fixing it.
A well-known example of persistent model uncertainty is aerosol radiative forcing of climate, for which the uncertainty range has remained essentially unchanged through all Intergovernmental Panel on Climate Change assessment reports since 1995. From the carbon cycle to ice sheets, each community will no doubt have its own examples.
We argue that the huge and successful effort to develop physical understanding of the Earth system needs to be complemented by greater effort to understand and reduce model uncertainty. Without such reductions in uncertainty, the science we do will not, by itself, be sufficient to provide robust information for governments, policy makers, and the public at large.
Model Wiggle Room“The scientist cannot obtain a ‘correct’ model by excessive elaboration.”As British statistician George Box famously said, “all models are wrong, but some are useful.” Less known is what Box said next: “The scientist cannot obtain a ‘correct’ [model] by excessive elaboration” [Box, 1976].
Most modelers would probably disagree that current model developments are excessive. But the point that Box was making is that models are only representations of reality and are therefore full of uncertain numbers, many of which cannot be defined experimentally. Even elaborate representations based on good process-based understanding are uncertain. In other words, our model simulations have an enormous number of degrees of freedom. We can think of this as wiggle room.
So how much wiggle room do we have in our models, assuming we have decided what the key processes are? In a model with, conservatively, 20 important and uncertain processes, each associated with a single uncertain parameter, the model outputs can be sampled from 20-dimensional space. This is a hypercube with around a million corners.
This complex space remains almost entirely unexplored because limited computational resources usually force research teams to settle on one “variant” of the model arrived at through tuning.
What We Lose When Settling on One Model VariantSelecting one model variant means that we never get to see many other perfectly plausible variants of the model, which may exhibit quite different behaviors when used to make predictions.
What is the problem with selecting one “best” model variant from the enormous set of potential variants? After all, we have drawn on years of experience and substantial resources at a model development center to select it.
The problem is that useful or not, one variant of a model tells us nothing about the uncertainty of the model predictions. To determine the uncertainty in the model predictions, we would need to identify all the plausible model variants, that is, those that can be judged as consistent with observations. But with so many degrees of freedom, there are, of course, a huge number of plausible model variants.
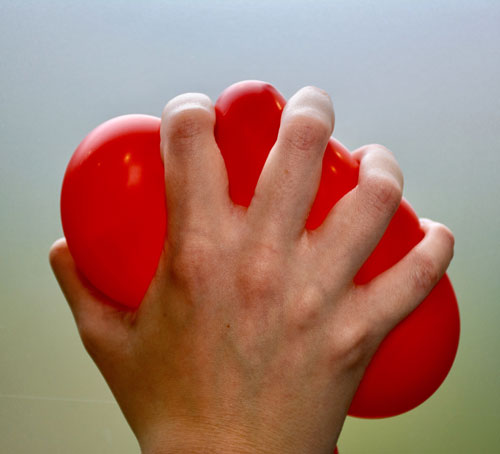
Every model developer recognizes the problem of trying to find a plausible model as the “balloon-squeezing problem.” Yes, we’ve squeezed the balloon at one point to generate a plausible model when judged against particular observations, but this creates a bulge of less plausible solutions somewhere else.
Model Intercomparison ProjectsModel intercomparison projects are the main way that the community explores some of the uncertainties. The idea is simple: Each model is like a differently shaped balloon, so comparing these models may reveal models’ strengths and weaknesses.
How useful is diversity across multiple models? Model diversity can tell us a lot about model realism when we discover unique or common model deficiencies. But when it comes to understanding and reducing uncertainty, we need to bear in mind that a typical set of, say, 15 tuned models is essentially like selecting 15 points from our million-cornered space, except now we have 15 hopefully overlapping, million-cornered spaces to select from.
Although these models are individually well chosen and form a “collection of carefully configured best estimates” [Knutti et al., 2010], they clearly aren’t a representative sample of all possible models. Your statistics instructor would tell you to go and collect more data before drawing any inferences.
The Dangers of Using a Small Set of ModelsWe need to be very wary of using a small set of models to observationally constrain model predictions.We need to be very wary of using a small set of models to observationally constrain model predictions.
An example of observational constraint (or model screening) is the multimodel Atmospheric Chemistry and Climate Model Intercomparison Project study [Shindell et al., 2013], in which five models were selected from nine on the basis of their skill in simulating aerosol optical depth. The five models had a smaller range of simulated aerosol radiative forcing than the nine. The authors were open about the procedure, noting that the set of screened models would probably be different if uncertainties in aerosol emissions had been accounted for.
But the emissions in this case were just one of many important uncertainties in the million-cornered hypercube. Without any estimate of uncertainty in the model predictions, such screening cannot provide a statistically robust estimate.
Many studies use emergent constraints to attempt to narrow the multimodel range. In this approach, a linear relationship between an unobservable variable of interest (like aerosol forcing or future temperature) and an observable variable (like aerosol optical depth or sea ice cover) simulated by multiple models is used to read off the “observationally constrained” value of the variable of interest. But with only a small set of models drawn from a potentially much larger set, there is a risk that such correlations may be artificially inflated, regardless of whether the relationship can be interpreted physically or not.
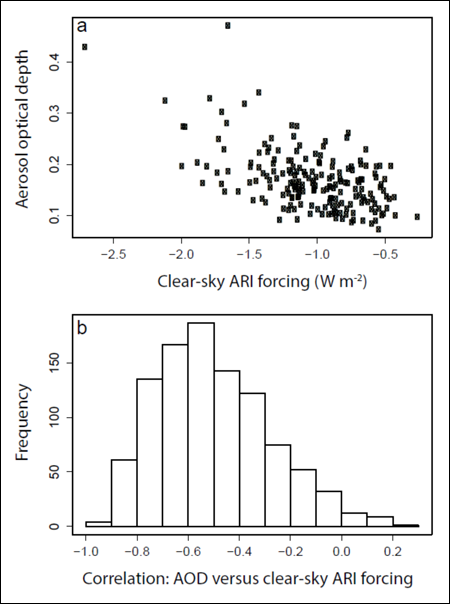
As an example, Figure 1a shows how the aerosol forcing correlates with optical depth in a set of 200 model simulations in which 27 model parameters were perturbed. The correlation coefficient is 0.55. Figure 1b shows the correlation coefficient for many samples of 15 models drawn randomly from the 200.
The example shows that there is quite a high chance of finding sets of 15 models with a linear correlation that is much higher than that of the entire population of models. If each model in a multimodel ensemble sampled the wider uncertainty space, our belief in the effectiveness of strong multimodel emergent relationships might be reduced.
A Way ForwardScientists appear to have no option but to carry on this way, despite evidence that our progress toward reducing climate model uncertainty is very slow and knowing that we are overlooking many uncertainties. Models with higher complexity will undoubtedly have greater fidelity for some problems, but it is doubtful whether such model “elaboration” will get us any closer to reducing the overall uncertainty. In fact, it may have the opposite effect [Knutti and Sedláček, 2012].
We suggest a different framework. If the scientific community wants to reduce uncertainty, then it needs to treat uncertainty as one of the primary scientific challenges and tackle it directly.
Alongside current approaches of model development and refinement (which we certainly don’t reject), we advocate that more needs to be done from a system uncertainty point of view. Many funding proposals and publications often have the stated aim of “reducing uncertainty,” usually by process elaboration, but the climate modeling community tends to treat model uncertainty as a secondary challenge and rarely plans strategically for its reduction.
However, there are some very useful statistical techniques that can generate model outputs across the million-cornered space based on a manageable number of training simulations. These and other statistical techniques are being applied successfully across a range of complex science problems as diverse as hydrology [Beven and Binley, 2013], disease transmission [Andrianakis et al., 2015], galaxy formation [Rodrigues et al., 2017], aerosol modeling [Lee et al., 2013], and, increasingly widely, climate science [Qian et al., 2016].
Once the model uncertainty has been comprehensively sampled using an arbitrarily large sample of model variants, some of the important sources of uncertainty can be made clear. It is then possible to screen models in a statistically robust procedure, such as through “history matching” [Craig et al., 1996]: seeing whether past real-world behavior matches model output. Structural deficiencies can even be detected by comparing with observations using a statistical framework [McNeall et al., 2016].
We feel that faster progress is much more likely if scientists integrate such uncertainty quantification approaches into wider model development and the intercomparison activities that underpin much of climate model assessment.
The Weight of Neglected UncertaintyIt would be a shame if the endeavors of 10,000 geoscientists led us to a point of exquisite model beauty, only to discover that these exquisite models are burdened by an accumulation of decades’ worth of neglected uncertainty.More robust statistical procedures are not straightforward or undemanding in terms of computing resources, nor are they the whole solution to the problem of developing robust climate models [Knutti et al., 2010]. Hundreds of simulations are required to build the statistical picture, and ever larger volumes of data need to be handled. It is unlikely that any more than a few modeling centers could commit to this approach.
But we need to weigh up how much effort should go into model “elaboration” versus efforts to tackle uncertainty. They are not the same problem.
It would be a shame if the endeavors of 10,000 geoscientists led us to a point of exquisite model beauty, only to discover that these exquisite models are burdened by an accumulation of decades’ worth of neglected uncertainty. At the very least, we won’t understand the uncertainty in a collection of elaborate models unless we make more effort to calculate it.
AcknowledgmentsThis research was partly funded by NERC grants NE/J024252/1 (GASSP), NE/I020059/1 (ACID-PRUF), and NE/P013406/1 (A-CURE) and by the U.K.–China Research and Innovation Partnership Fund through the Met Office Climate Science for Service Partnership (CSSP) China as part of the Newton Fund. L.A.L. was funded by a Leverhulme Fellowship.
from Eos https://eos.org/opinions/climate-models-are-uncertain-but-we-can-do-something-about-it?utm_source=rss&utm_medium=rss&utm_content=climate-models-are-uncertain-but-we-can-do-something-about-it
via IFTTT